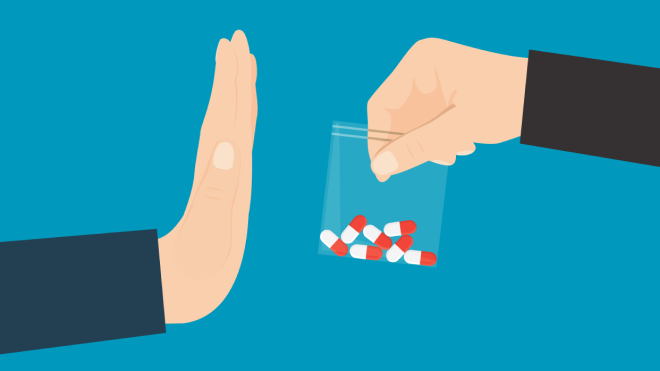
02 Nov Illicit Synthetic Drugs: AI to track evolution
Tracking synthetic illicit drugs production and their traffic online is a challenging job due to the fast pace with which the market evolves. Expert.ai has designed and developed a module for the automatic learning of new knowledge.
New scientific papers, which expose chemicals as new precursors to synthesize drugs are regularly published:
“Instead of importing the prohibited but necessary chemicals, offenders started to produce these chemicals themselves, resulting in the use of pre-precursors (such as safrole for PMK and APAAN for BMK). A side effect of this methodical displacement was that it became possible to produce significantly cheaper chemicals, which could result in much cheaper ecstasy.” [E.R. Kleemans & M.R.J. Soudijn (2017). Organised crime. In: N. Tilley and A. Sidebottom (eds.). Handbook of Crime Prevention and Community Safety. Second edition. Routledge]
From this scrap, we know in advance that PMK and BMK are used to produce ecstasy,
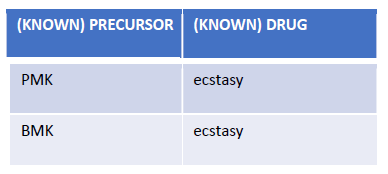
Image 1: Known relations between precursors and drugs
so we look for markets that sell these products to discover producers of ecstasy. Also, there are other substances named in the paper that are not contemplated by the knowledge base (safrole and APAAN). In cases like these, the NLP algorithms developed by Expert.ai are capable of identifying these new chemicals of interest starting from the context. Upon the validation of experts, new knowledge is automatically identified, learned, and acquired by the system.
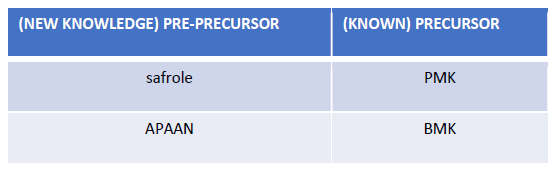
Image 2: Discovered relations between pre-precursors and precursors
The ability to use AI techniques to automatically gain new knowledge about the latest techniques to produce illicit substrates is of great aid to LEAs who would otherwise spend a lot of valuable resources not to be outpaced by the fast evolution of the illicit synthetic drugs production.
New knowledge recognition has been tested to calculate its performance in terms of precision and recall. Also, here the process was manual with a non-tagged corpus retrieved by Expert.ai’s crawler, but it was led before the work of verticalization of the Expert.ai’s semantic network (Sensigrafo), in order to find as much new entities and relations as possible. The documents were 18, containing a total of 54 new entities and relations.
The first analysis made by the new knowledge algorithms for entities retrieved 20 true positives, six false positives, and 16 false negatives. The first 20 new entities have been validated by investigators; a second round of analysis detected other six true positives as pre-precursors.
Finally, the last round of analysis was led with New Knowledge algorithms for relations, which were capable of re-tracing all the relations pertaining to all the new entities retrieved in the first two rounds, for a total of 20 + 6 true positives. The six false positives returned in the first analysis were discarded and so, they would not enter the KB, thus disappearing from further rounds of analysis. The 16 false negatives were instead maintained.
The result is an encouraging and promising score, since it translates in 81 out of 100 useful new entities and relations, Recall 62%, and F-Measure 70%.
Author: Ciro Caterino, Expert.ai
References:
Masucci, V., Colombo, J., Caterino, C. and Nardelli, F. (2021) “ANITA’s Text Analysis Services for Fighting Online Illegal Trafficking of Drugs, Weapons and False Charity Claims: A Lateral Thinking Approach” 2021 NBP 2021, Vol. 26, Issue 3, pp. https://doi.org/10.5937/nabepo26-33849.
ANITA – Newsletter 6. Available at: https://www.anita-project.eu/newsletter.html