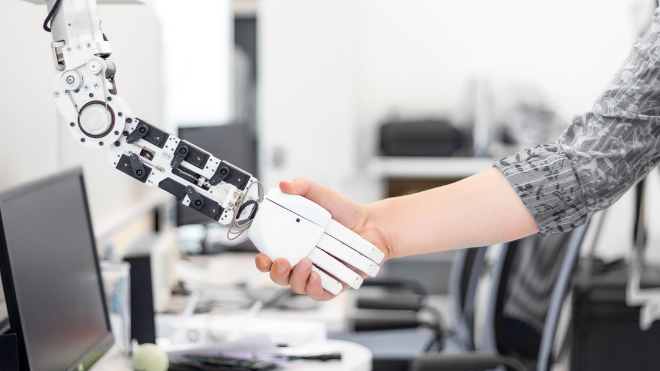
26 Jan Trustworthiness of the AI: what is to be faced
As the participation of AI expands in areas such as transportation, finance, medicine, security and entertainment, society is realizing the high importance of the Trustworthiness of the Artificial Intelligence as a Service. Lack of stakeholder confidence in such systems is a significant problem hindering their effective use in everyday life.
To evaluate the attractiveness of such systems, one should take into account their various aspects, such as their reliability, algorithmic fairness, explainability and transparency.
While the most active academic research into AI reliability has focused on the algorithmic properties of models, advances in algorithmic research alone are not enough to create robust AI products. From an industrial point of view, the life cycle of an AI product consists of several stages, namely:
- data preparation,
- development of an algorithm,
- development and deployment
- exploitation,
- monitoring
The reliability of AI must be established and systematically assessed throughout the life cycle of an AI system.
Improving reliability in any single aspect requires efforts at several stages of this life cycle, such as cleaning data, using robust algorithms, monitoring anomalies, and auditing risks.
Individual improvement of each aspect of reliability does not guarantee a more reliable and efficient end result. In this regard, a coordinated cumulative optimization of several aspects of reliability simultaneously is necessary.
A new approach is needed to move artificial intelligence technologies from the performance plane to the reliability plane. This requires conscientiousness on the part of representatives of various disciplines and interdisciplinarity and cooperation when working on various aspects of reliability and at different stages of the system life cycle.
Author: Tetyana SOLIANYK
Reference:
Tsipras et al. “Robustness May Be at Odds with Accuracy” (2019)
Bodria et al. “Benchmarking and Survey of Explanation Methods for Black Box Models”. arXiv:2102.13076 (2021).