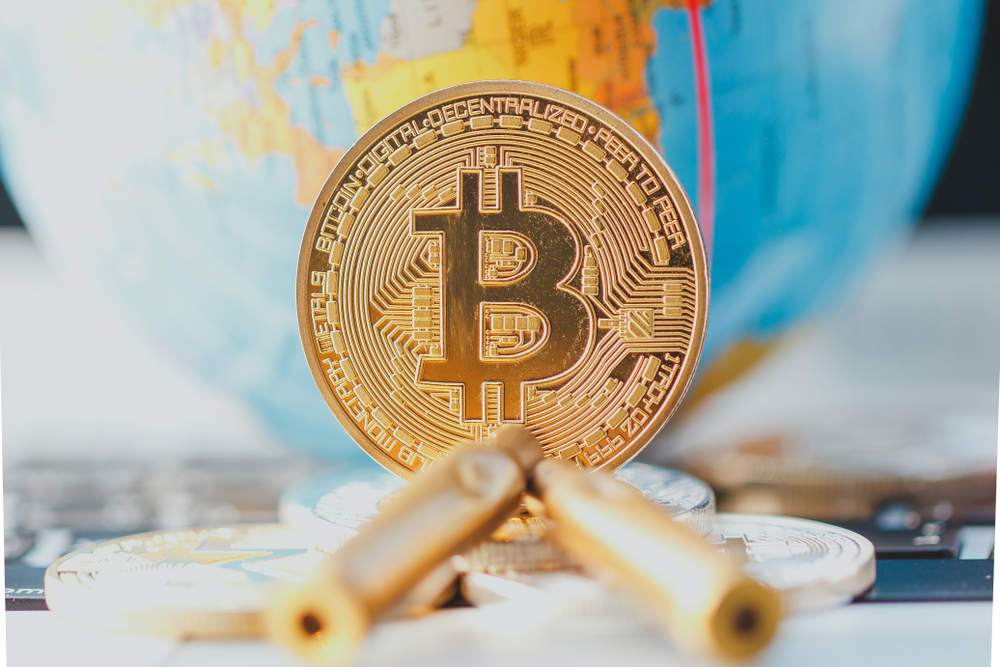
24 Apr Role of blockchain intelligence for preventing money laundering and terrorism financing
This paper gives review on possible intelligence levels what Law enforcement agencies can provide on top of blockchain data. New technologies bring new challenges. Criminals are using mor sophisticated methods by performing cross chain transactions, changing cryptocurrency to other values like Monero or Zcash which provides better anonymity. It is hard to distinguish legitime transactions from illicit ones which brings lot of falls positives and it can cost lot of resources and time without good result. Problem is that still lot of Law enforcement agencies don’t have enough experience and right tools to deal with blockchain technologies. That why Law enforcement agencies has joined with academics and other practitioners to improve their knowledge about most recent technologies. Project iNteracting netwOrk of inTelligence and securIty practitiOners with iNdustry and acadEmia actorS (NOTIONEs) which is funded H2020 – Coordination and Support Action (CSA) has several working groups about important topics and one of these working groups focusing on cryptocurrency tracing possibilities.
Introduction
Nowadays global economy has faced huge number of technological innovations in financial markets. Regular introduction of new forms of payment services and service providers happening all the time, such methods as mobile payments, or payment on e-mail, Mobile Point of Sale (mPOS) becoming more and more popular. Mobile Finance Apps report shows that Users already in 2020 installed 4.6 billion finance apps globally and spent 16.3 billion hours in-app (Liftoff, 2020), which shows how active market are in electronic world.
Important part of technological innovations are connected with rise of virtual currencies. According to CoinMarketCap, the value of all the bitcoins in the world was over $1.03 trillion as of Nov. 26, 2021 (CoinMarketCap, 2021). The same time, bitcoins are not only cryptocurrencies, in fact there are more than thousand different crypto assets or even more. However, as more parties enter these markets, criminals trying exploit vulnerabilities to launder illicit funds and finance terrorism. Money laundering (ML) can be represented as process of legitimizing criminal proceeds and often it is combined with terrorism financing (TF) which is any form of financial action for terrorist group financing (Teichmann, 2019), that why Financial Intelligence units (FIU) in each country trying to analyze various date to prevent exploitation of financial instruments and also new technologies for ML/TF purposes.
FIUs together with regulators trying to combat ML/TF by implementing measures and standards by implementing traditional ‘know your customer’ (KYC), customer due diligence (CDD) and transaction monitoring to virtual asset service providers (VASPs). For example FATF (The Financial Action Task Force) have set recommendations which basically are standards for member countries and financial institutions which provide strategies to detect ML/TF activities. Unfortunately, often not VASPs are not provide accurate KYC policy and there is still lack of common understanding about VASPs requirements and their duties. For example, in Latvia 10 legal entities has registered as VASPs, but In the second half of 2022, no suspicious transaction reports from VASPs have yet been received by the FIU (Latvia, 2022). If we compare it with traditional financial institutions than reports from these institutions come regularly. Also at the EU level, work for common legislation has been underway since Q3 2020 on the MiCA single framework, which will replace national regulations on virtual assets once it is developed. This will require national regulation to transpose the scope and requirements of the MiCA Regulation. The MiCA regulation will provisionally enter into force just in 2025.
This shows that Law enforcement agencies and FIU has to work proactively and try to use some tools to monitor suspicions transactions which is connected with virtual asset transactions and try to react if illegal activities have been noticed. Important part of successful investigations are possibilities to provide qualitative analysis and provide intelligence on top of available data. That why aim of this paper is to study role of intelligence of crypto asset monitoring and provide overview of possible work directions to improve Law enforcement agencies work on crypto asset transaction analysis.
Block chain intelligence levels for cryptocurrency analytics
Cryptocurrencies based on blockchain technology which is peer-to-peer network and serves as public ledger. Blockchain transactions are publicly available for reading, but these transactions and their records can’t be modified. Blockchain stores information across nodes and distributes changes to all involved parties. Cheating or faking some records in this system are practically impossible, because entire network looking after it. That why blockchain technology could be considered more transparent than banking system. And blockchain is not just criminal money exchange platform, it can be used in healthcare, IoT, legal perspective, government, power grid, Transport system, commercial world, cloud computing, reputation, E-business and other areas (Bhabendu Kumar Mohanta, 2019).
Problem is that actors behind transactions and wallets are encrypted in hash values, so to track these hash values and connect them with real persons is not so easy, especially if VASPs do not provide accurate KYC policy, but the same time size and trace of virtual currencies continue to grow. For analyzing crypto transactions and perform “follow-the-money” process there are several Blockchain explorers” which are publicly available. Such tools which allow analyze raw blockchain data emerged in 2010, soon after the lunch of Bicton blockchain, providing possibility to retail investors look up their own cryptocurrency transactions, but these tools can be also used for investigation purposes as well. Such tools can be found in URLs like https://www.blockchain.com/, https://oxt.me/, https://www.walletexplorer.com/, etc.
Off course dealing with raw data is slow process and requires lot of effort analyze such amount of information. Therefore, blockchain analytics have grown already to next level and started to combine raw data with a proprietary database of known addresses to link activities of blockchain world with activities in real world. Such database allows to label bad actors, make risk scores, connect addresses with certain VASPs or some darknet activities. Information storage about transactions also provides possibility determine the list of entities that the address or some other party interacts with, link nodes with IP addresses. Unfortunately such storage and analytics also requires lot of resources. One think is storage itself, but it requires data analysts and developers to implement intelligence on top of these data. And it must be done regularly, because each day happening lot of transactions, some new addresses showing up in criminal activities and it must be traced, mixed with OSINT data and other sources, so that it can be marked and used for investigations. Most of cases these activities already are performed by commercial tools like CipherTrace[1], or others.
In ML/TF cases it is important to follow money, to understand full case and also find out what kind of value are created with illicit money. That why nowadays it is not enough with actor labeling, but it is necessary to draw money flow from one point to another. Which brings already to another level of intelligence where it is possible to trace crypto transactions between multiple blockchain schemas. Due transparency of transactions in blockchains like Bitcoin, criminals trying to hide their identity by performing cross-chain changes of crypto assets, often shifting to alternative currency types like Ethereum, Monero or Zcash because of anonymity and privacy options these cryptocurrencies may provide (Europol, 2017).
In that case it necessary to go beyond simple labeling, “black listing” and string matching. In this level it is necessary to dynamically recognize certain patterns which indicates possible money laundering or same fraudulent activities. Pattern matching often used in IT security to recognize intrusion detection or possible malware. Such analytics allows recognize possible clusters, nested transactions, groups of transactions which could be connected with money laundering, trace funds from source to destination or other schemes. For such level it is necessary to use already even more sophisticated tools like TRM-Labs[2] or Chainalysis[3].
Last few years tremendous attention has received machine learning. Data mining and machine learning could be final layer for blockchain analysis. Cryptocurrency transactions could be counted as big data, especially if it has combination with OSINT data. Machine learning methods allow explore the patterns and recognize suspicions transactions, possible financial fraud schemas or other risk areas. For such data analytics following methods could be effective to work in financial data domain:
- Support vector machine (SVM) – the objective of the support vector machine algorithm is to find a hyperplane in an N-dimensional space(N — the number of features) that distinctly classifies the data points(Gandhi, 2018). This method has been proposed to monitor bank account flow (Xue-Zhi QIN, 2014) and also for improving money laundering detection (B. N. Pambudi, 2019). There is also study about SVM usage for transaction categorization in fraudulent and legitimate transactions by using credit card dataset (V. Mareeswari, 2016).
- Bayesian network (BN) – are networks with connected variables by creating form of graphical model and showing relationships between different variables. This method often is used when there is a lack of data available, it is helpful for searching probabilities in the existence of uncertainty. Saleha Raza and Sajjad Haider showing that dynamic Bayesian networks can be useful in anti-money laundering domain for detection anomalies in sequence of transactions(Saleh Raza, 2011) . There is another research which propose intelligent detection focusing on fraudulent financial reports (Peter Hajek, 2017). Authors experimented with variables and several other methods, but BN outperformed other machine learning methods.
- K-Nearest Neighbor algorithm (KNN) – this method trying to find closest neighborhoods based on similarity from a given dataset and generate a new sample point based on the distance measure between two data samples. This method often used for credit card fraud detection, but also can be used to detect illicit transactions in Bitcoin blockchains(Abdelaziz Elbaghdadi, 2021). Study materials show that this method mostly used for simple recommendation systems, pattern recognition, data mining, financial market predictions, intrusion detection, and more.
- Decision tree (DT) – Decision tree algorithm is the most widely used method in data mining classification algorithms. It is a classification tree utilized to create a decision support tool between nodes over the features and dependencies which represents result of selection. Logistic regression is commonly used for problems where the input data is comprised of multiple variables and when there are many outliers in the data set. Decision tree is simple to understand and to interpret and is readily visualized. Outputs can be easily motivated with simple Boolean logic. Currently there are multiple articles which show DT connection to anti money laundering risk evaluation(Vikas Jayasree, 2017).
- Fuzzy logic-based system – this method provides possibility to complicated modeling for handling data in vague and inaccurate environment. Research form Y. Chen and J. Mathe shown that with fuzzy computing it was possible to discover that one party controlling multiple accounts, from existing fraud models could be isolated fraudsters (Yo-To Chen, 2011). There is also another research which shows that fuzzy logic by fallowing Neural-fuzzy Takagi-Sugeno training method coul’d be found fraudulent banking transactions (F.S. Nezhad, 2013).
Evaluation of several machine learning methods showing that there is great potential to improve data analysis on top of financial fraud, ML/TF, and also cryptocurrency fraud. At the moment more popular are supervised learning techniques of machine learning than unsupervised techniques. From research evaluation it is possible to see that supervised learning showing better performance. Mentioned algorithms are not only one’s who showing up there are also methods like – Hidden Markov model, artificial neural networks, genetic algorithms and others, which showing that Artificial intelligence can bring extra value to anti money laundering and fraud detection in financial activities and also in cryptocurrency transactions.
Conclusion
Blockchain is new and important technology which can make changes in wide variety of industries. Unfortunately, big part of crypto transactions are used for criminal activities. Even if analytical methods become more effective and much faster, cryptocurrencies continue evolve their privacy possibilities and try to hide real identity of end user. Currently there are lot of commercial tools who propose possibilities to analyze blockchain transactions and also trying themselves to make registers of suspicions wallets and VASPs and more advanced tools even allow follow money flow between multiple block chains. Important part that such analytics require not just good tools, but also good understanding how these transactions work and how to use that information. Positive part that lot of commercial tools also providing certifications and training which allows to use these tools more effectively. Anyway tendencies show that Law enforcement more and more will need to shift from simple case analytics to in-depth data research and intelligence.
[1] https://ciphertrace.com/
[2] https://www.trmlabs.com/
[3] https://www.chainalysis.com/
Author(s): Nauris Pauliņš Financial Intelligence Unit Latvia (FIU)
Reference:
Transaction in the Bitcoin Network. Integration Challenges for Analytics, 18.N. Pambudi, I. H. (2019). Improving Money Laundering Detection Using Optimized Support Vector Machine. International Seminar on Research of Information Technology and Intelligent Systems (ISRITI), 273-278.
Bhabendu Kumar Mohanta, D. J. (2019). Blockchain technology: A survey on applications and security privacy Challenges. Elsevier, 19.
CoinMarketCap. (2021). Bitcoin. Ielādēts no https://coinmarketcap.com/currencies/bitcoin/
Europol. (2017). Internet Organised Crime Threat Assessment. Hague.
F.S. Nezhad, H. S. (2013). Fuzzy logic and Takagi-Sugeno Neural-Fuzzy to Deutsche bank fraud transactions. E-Commerce in Developing Countries: With Focus on E-Security, 1-15.
Gandhi, R. (2018). Support Vector Machine — Introduction to Machine Learning Algorithms. Ielādēts no https://towardsdatascience.com/support-vector-machine-introduction-to-machine-learning-algorithms-934a444fca47?gi=33c63625690b
Yo-To Chen, J. M. (2011). Fuzzy Computing Applications for Anti-Money Laundering and Distributed Storage System Load monitoring. World conference on soft computing .
Latvia, F. I. (2022). Virtual Assets: Money Laundering and Terrorism and Proliferation Financing Risk Assesment. Riga.
Liftoff. (2020). 2020 Mobile App Trends Report. Ielādēts no https://content.liftoff.io/hubfs/
Peter Hajek, R. H. (2017). Mining corporate annual reports for intelligent detection of financial statement fraud – A comparative study of machine learning methods. Knowledge-Based Systems, 139-152.
Saleh Raza, S. H. (2011). Susspicious avtivity report using dynamic bayesian networks. Proceda Computr science, 987-991.
Teichmann, F. M. (2019). Recent trends in money laundering and terrorism financing. Journal of Financial Regulation and Compliance, 12.
4.Mareeswari, G. G. (2016). Prevention of credit card fraud detection. Information Communication and Embedded Systems, 4.
Vikas Jayasree, R. S. (2017). Money laundering regulatory risk evaluation using Bitmap Index-based Decision Tree. Journal of the Association of Arab Universities for Basic and Applied Sciences, 96-102.
Xue-Zhi QIN, J.-Y. L.-Q. (2014). SVM-based Abnormal Account Monitoring Model of Bank. International Conference on Economic Management and Trade Cooperation, 8.