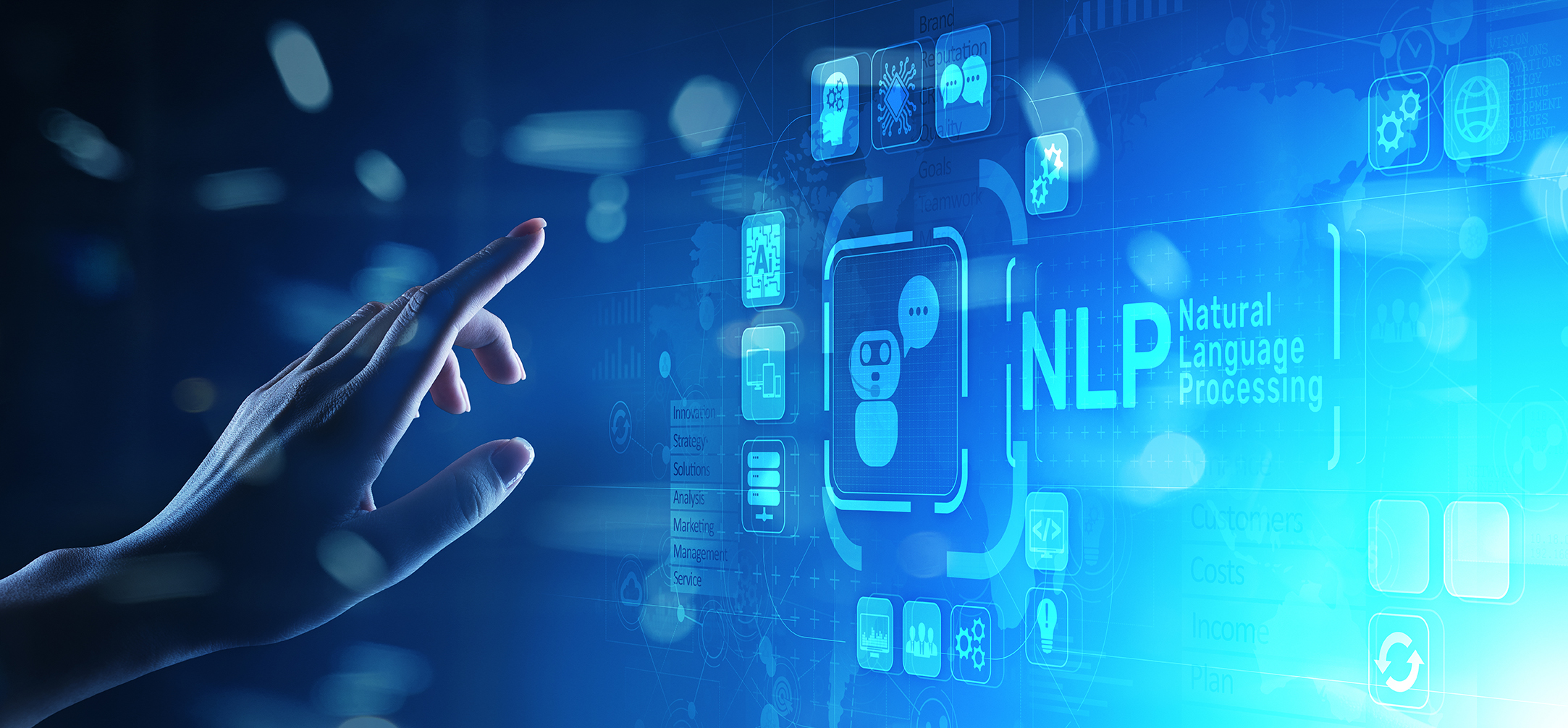
20 Mar Expert.AI supports Intelligence activities: exploring features of and differences among NLP, NLU, NLG
Introduction
The intelligence activities in the field of the fight against criminality and terrorism include the capability of recognizing signals and facts of interest within unstructured textual contents.
The possibility of retrieving information contained in proclaims, disseminations, propaganda in general, is a very useful instrument that can help the intelligence agencies to prevent and to implement countermeasures to illegal activities.
With this purpose, Expert.AI has recently pointed out the most important features of and difference among NLP, NLU, NLG.
What is NLP?
Natural Language Processing turns words into action. For machines, human language, also referred to as natural language, is how humans communicate—most often in the form of text. This format is not machine-readable, and it’s known as unstructured data. It comprises the majority of enterprise data and includes everything from text contained in email, to PDFs and other document types, chatbot dialog, social media, etc.
A subfield of artificial intelligence and linguistics, NLP provides the advanced language analysis and processing that allows computers to make this unstructured human language data readable by machines. It can use many different methods to accomplish this, from tokenization, lemmatization, machine translation and natural language understanding.
What is NLU?
For a person to learn a language, it can take 2,000 hours or more. Grammar complexity and verb irregularity are just a few of the challenges that learners encounter. Now, consider that this task is even more difficult for machines, which cannot understand human language in its natural form. NLU Creates order out of chaos.
Instead, machines must know the definitions of words and sentence structure, along with syntax, sentiment and intent. Natural language understanding (NLU) is concerned with the meaning of words. It’s a subset of NLP and It works within it to assign structure, rules and logic to language so machines can “understand” what is being conveyed in the words, phrases and sentences in text.
Natural language understanding uses a range of techniques (parsing and sentence analysis, grammatical and logical analysis, and finally semantic disambiguation) so as to provide a machine with the ability to truly grasp what’s happening in a document: who did what, to whom, where, etc. This allows it to disambiguate in other words, to understand ambiguity (meaning in context), to provide comprehension of text (versus just reading), and to understand semantics (meaning) and relationships between words in text.
NLU vs NLP: What’s the Difference?
Together, NLP and NLU are a powerful combination that can be used to transform unstructured data into information that can be leveraged for insight, intelligence, efficiency and automation for several real-world applications and use cases.
To differentiate between NLP and NLU, remember that:
- While both NLP and NLU focus on human language, their objectives are different.
- NLU fills the gap between human language and machine understanding.
- Where NLP breaks down language into a machine-readable format and processes language, NLU provides language comprehension.
- NLU learns language syntax, context, patterns, definitions, sentiment and intent.
What is NLG?
Where NLP helps machines read and process text and NLU helps them understand text, NLG or Natural Language Generation helps machines write text.
The “suggested text” feature used in some email programs is an example of NLG, but the most well-known example today is ChatGPT, the generative AI model based on OpenAI’s GPT models, a type of large language model (LLM). Such applications can produce intelligent-sounding, grammatically correct content and write code in response to a user prompt.
The ability of an application to be able to generate text depends largely on two things: the underlying AI approach for processing and training data and the dataset it is trained on. Therefore, while a NLG application is able to produce text that sounds correct, it does not mean that the text it produces is accurate or factual.
In the case of ChatGPT or any application trained on a large language model—with 45 terabytes of data in the case of GPT-3—it is trained using a machine learning approach that uses statistics and pattern matching to predict the words and phrases that come next. This is in contrast to NLU, which applies grammar rules (among other techniques) to “understand” the meaning conveyed in the text.
This difference is very important to distinguish when it comes to NLG and generative AI because the text it produces can be so authoritative and correct sounding that it’s easy for users to be convinced that it actually does understand the text that it is repeating.
To understand how NLG works, it’s important to remember that:
- NLG does not understand the text on its own—it needs the language rules and knowledge base integration with NLP and NLU to do that.
- The text that an NLP application produces is the result of pattern matching, not an understanding of facts.
- The text that NLG applications are able to produce depends on the data set that the underlying model/algorithm has been trained on.
Author(s):
Ciro Caterino, Marketing Dep., Expert.ai
Source(s):
Expert.ai Team (2023), Understanding NLP vs NLU vs NLG, https://www.expert.ai/blog/dont-mistake-nlu-for-nlp-heres-why/?, (Accessed on 19.03.2024)