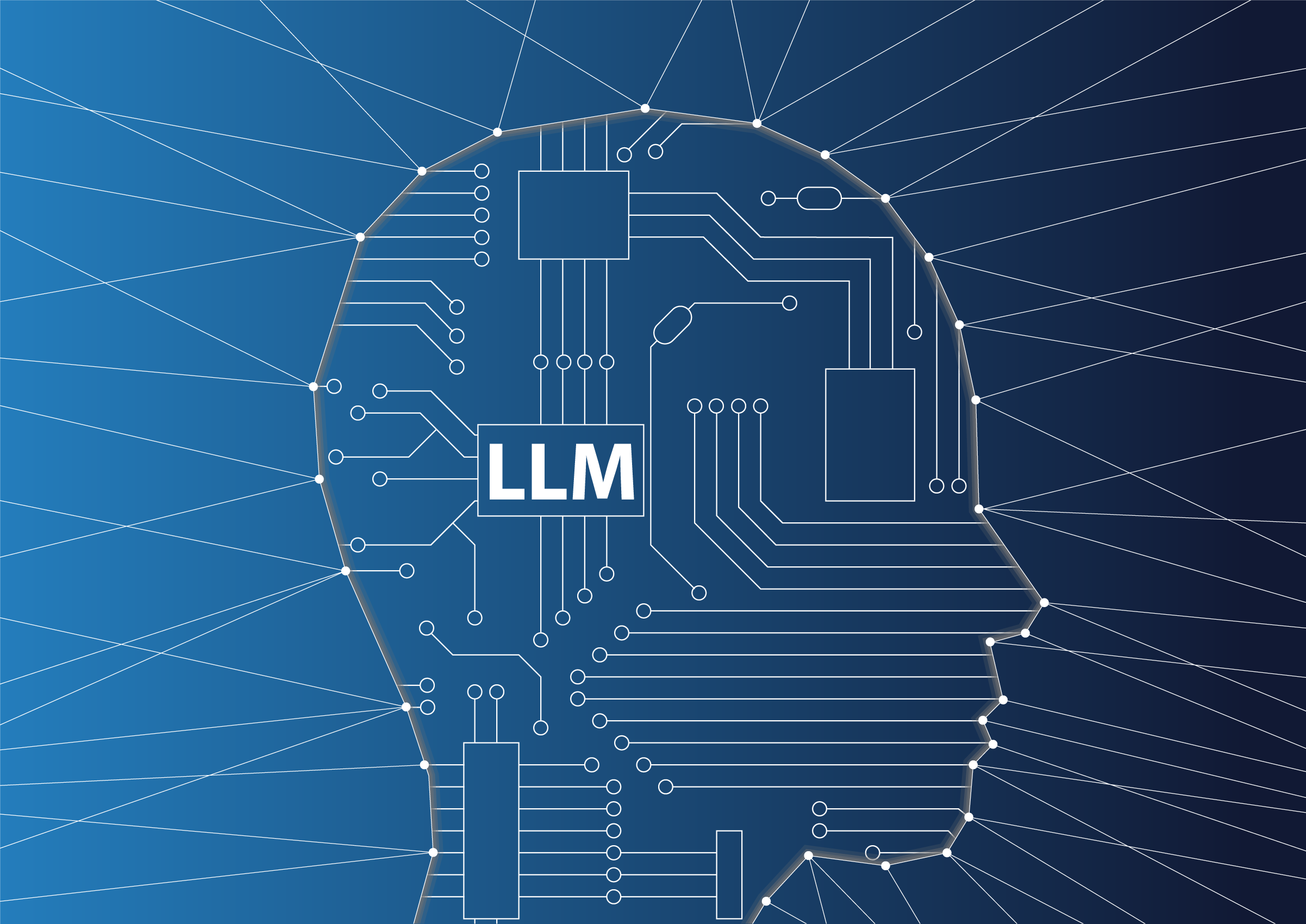
15 Apr Are LLMs dangerous? The case of ChatGPT in Italy and EU
Since ChatGPT was released in November 2022, it has created a lot of buzz in tech circles, but also in the mainstream. It has set companies racing to get their latest (and usually largest, although that is starting to change) LLMs to market, from Microsoft, to AWS, Google to Facebook and a number of open-source models. Those experimenting with ChatGPT range from middle school teachers to company CEOs, and the combined momentum has caused a lot of hype and concern around the benefits versus its potential for harm.
The criticism ramped up last week over a series of events. Recently, AI experts, industry leaders, researchers and others signed an open letter calling for a six-month “pause” on large-scale AI development beyond OpenAI’s GPT-4. The next day, AI policy group CAIDP (Center for AI and Digital Policy) launched a complaint with the FTC, arguing that the use of AI should be “transparent, explainable, fair, and empirically sound while fostering accountability,” and that OpenAI’s GPT-4 “satisfies none of these requirements” and is “biased, deceptive, and a risk to privacy and public safety.” Finally, on Friday, the first major chip to fall was the call by Italian regulators for OpenAI to address certain privacy and access concerns around ChatGPT.
As NOTIONES is dealing with projects and technologies that have or could have a great impact in the fields of security and intelligence activities, it is very useful starting to think about the usage and the weakness that LLMs could show regarding illegal threats.
In particular, main answers that humans (and Intelligence agencies!) should look for regard the following questions:
- Can it be ensured that criminality and terrorists will not be able to exploit the LLMs for illegal activities?
- Are the LLMs safe against possible data-breach?
- Is it possible to implement worldwide shared policies in order to avoid that a given government/state could exploit the LLMs for controlling people?
Why did Italy “Ban” ChatGPT?
Natural Language Processing turns words into action. For machines, human language, also referred to as natural language, is how humans communicate—most often in the form of text. This format is not machine-readable and it’s known as unstructured data. It comprises the majority of enterprise data and includes everything from text contained in email, to PDFs and other document types, chatbot dialog, social media, etc.
A subfield of artificial intelligence and linguistics, NLP provides the advanced language analysis and processing that allows computers to make this unstructured human language data readable by machines. It can use many different methods to accomplish this, from tokenization, lemmatization, machine translation and natural language understanding.
Considerations for AI Applications Going Forward
The concerns being raised are a reminder of the risks of some types of AI, but it’s also a time to reflect on the proven AI capabilities already at work. Expert.AI has delivered over 300 natural language AI solutions over the past 25 years, working with many Fortune 2000 companies to optimize processes and improve the work humans do. Expert.AI doesn’t just insist on having a human in the loop, Expert.AI works to humanize the work that is done – to make it more engaging and have humans add value to the solutions.
In that vein, Expert.AI wants to share some general considerations around using any AI to solve the real-world business problems.
1. Transparency and explainability should be built into any AI solution
Large language models like GPT-3 and GPT-4 are so large and complex that it is the ultimate “black box AI” approach. If you cannot determine how an AI model arrived at a particular decision, this could eventually be a business problem and, as we are seeing play out now, a regulatory problem. It’s absolutely critical that the AI you choose is able to provide outcomes that are easily explainable and accountable.
The path to AI that solves real-world problems with the highest degree of accuracy is through a hybrid approach that combines different techniques to take advantage of the best of all worlds.
Symbolic techniques leverage rules and, in the case of expert.ai, a rich knowledge graph — all elements that are fully auditable and understandable by humans. When paired with machine learning or LLMs, these combined techniques introduce much needed transparency into the model, offering a clear view on how the system behaves in a certain way to identify potential performance issues, safety concerns, bias, etc.
2. The data you use matters
The data you choose to train any AI model is important, whether you’re working with an LLM, machine learning algorithms or any other model.
Public domain data such as the data used to train ChatGPT is not enterprise-grade data. Even if the content ChatGPT has been trained on covers many domains, it is not representative of what is used in most complex enterprise use cases, whether vertical domains (Financial Services, Insurance, LifeSciences and Healthcare) or highly specific use cases (contract review, medical claims, risk assessment and cyber policy review). So, even for chat/search use cases—the ones that work similarly to ChatGPT—it will be quite difficult to have quality and consistent performance within highly specific domains.
The very nature of the data that ChatGPT has been trained on also creates concerns for copyright infringement, data privacy and the use and sharing of personally identifiable information (PII). This is where it comes up against the European Union’s GDPR and other consumer protection laws.
Natural language AI is most useful when it is built on, augments and captures domain knowledge in a repeatable way. This requires engineering guardrails (like the combination of AI approaches Expert.AI uses in Hybrid NL), embedded knowledge and humans in the loop. We built our platform on all three of these pillars for that reason and because it allows businesses to build accretive and durable competitive advantage with their AI tools.
3. A human-centered approach is critical
Having humans at only the beginning or only the end of an AI process is not enough to ensure accuracy, transparency or accountability. Enterprises need a human-centered approach, where data and inputs can be monitored and refined by users throughout the process.
Only explainable-by-design and interpretable-by-design AI models offer humans full control during the development and training phases. Because it includes an open, interpretable set of symbolic rules, hybrid AI can offer a simpler way to correct insufficient performance. So, if an outcome is misleading, biased or wrong, users can intervene to prevent future mistakes and achieve the success metrics most valuable for each use case and improve accuracy, all by keeping a human subject matter expert in the loop.
Whereas black box machine learning models only offer the opportunity to add more data to the training set without an opportunity to interpret the results, a hybrid approach can include linguistic rules to tune the data in the model. Hybrid AI makes it possible for people to be in control of a model.
The end result should also include an analysis of not just the ROI provided but the human benefit. Did menial or redundant tasks get automated and is there value in the solution for the humans receiving the benefits? While ROI, consistency and automation are typical results in any AI project, those including natural language solutions often provide additional upside. The work that humans do is more engaging, critical and rewarding. In combination with humans in the loop, this is human-centered AI.
Author: Ciro Caterino, Marketing Dep., Expert.ai
Sources: Expert.ai (2023), https://www.expert.ai/blog/llm-hype-and-concern-benefits-versus-harm/, (Accessed on 15.04.2024)